Prediction of avalanche potential using convection-permitting meteorological model and snowpack model
Heavy snowfall is typically “concentrated”. It is necessary to predict or nowcast the potential of hazards caused by the heavy snowfall in meso-γ scale or smaller resolution. Researches to cope with this problem are conducted in the SIRC
NIED Snow Disaster Forecasting System (SDFS)
Models for the SDFS and data flow.Models for the SDFS and data flow.d data flow.Models for the SDFS and data flow.on of operational meteorological forecasts has improved significantly in recent years. This has enabled us to generate snow disaster information through appropriate modeling of snow processes. The SIRC/ NIED has constructed the Snow Disaster Forecasting System (SDFS) to predict avalanche potential, visibility in the blowing snow, and snow conditions on the road. We started from basic experiments on the formulation of physical processes, such as snow metamorphism and the development of blowing snow layers. New schemes, such as the dry snow metamorphism factor (DSM factor) describing snow grain evolution and the ejection factor for blowing snow particles were introduced and incorporated into the SDFS.
At the same time, we invested a great deal of effort in coupling a meteorological model of ~1-km resolution and snowpack and snow disaster models of ~10- m resolution (or for point prediction). We introduced the Japan Meteorological Agency nonhydrostatic model (JMANHM) for the prediction of snowfall. Currently, all models are connected and can be executed automatically when the input data are received. The SDFS has experimentally provided predictions to registered users in real-time.
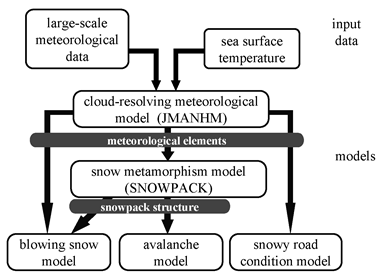
- Nakai, S., T. Sato, A. Sato, H. Hirashima, M. Nemoto, H. Motoyoshi, K. Iwamoto, R. Misumi, I. Kamiishi, T. Kobayashi, K. Kosugi, S. Yamaguchi, O. Abe, and M. Ishizaka, 2012: A Snow Disaster Forecasting System (SDFS) constructed from field observations and laboratory experiments. Cold Regions Sci. Tech., 70, 53-61.
- Nakai, S., K. Kosugi, S. Yamaguchi, K. Yamashita, K. Sato, S. Adachi, Y. Ito, M. Nemoto, K. Nakamura, H. Motoyoshi, H. Hirashima, I. Kamiishi, K. Oda, M. Ishizaka, O. Abe, and T. Sato, 2019: Study on advanced snow information and its application to disaster mitigation: An overview. Bull. Glaciol. Res., 37S, 3-19, doi:10.5331/bgr.18SW01.
Snowpack simulation
The SNOWPACK model is the main core of the avalanche prediction system, a component of the Snow Disaster Forecasting System. Input data for SNOWPACK are derived from meteorological observation data, mainly obtained from the SIRC and the Japanese Meteorological Agency (JMA). The SNOWPACK model requires inputs of air temperature, relative humidity, wind speed, wind direction, solar radiation, longwave radiation, precipitation, and snow depth. Although some of the input data may be directly taken from observations, some parameters must be estimated indirectly. If snow depth data are available at a meteorological station close to the simulated location, snowfall amount is estimated from snow depth. However, owing to high spatial variation in snow depth with elevation and orientation, precipitation is usually used to derive snow depth at the avalanche slope.
The SNOWPACK model is a one-dimensional model predicting the various physical parameters of layered snowpack. The surface meteorological variables from JMANHM output and SW-Net observations of the NIED are used as inputs of the SNOWPACK model in the SDFS (Nakai et al., 2012). The SNOWPACK model is used to evaluate and predict the characteristics of each layer of snowpack, e.g., grain type, grain size, density, temperature, liquid water content, and shear strength.
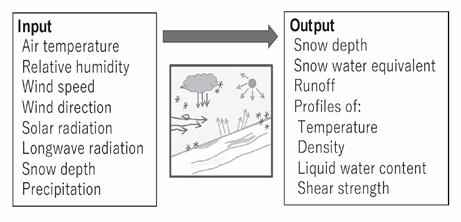
(Hirashima et al., 2020)
The JMANHM, which is operated in a resolution of 1.5 km by NIED, is used to provide 30-hour-long meteorological forecast data beginning at 09 and 21 UTC daily. The JMANHM outputs for 0-20 m elevation provide all of the meteorological parameters necessary for input into the SNOWPACK model.
Simulation is updated for every three hours replacing forecasted data with latest observed data. Simulated results were provided to registered users experimentally such as local governments and road administrators.
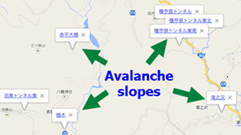
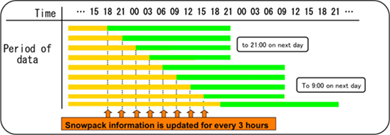
Interpretation of simulation results
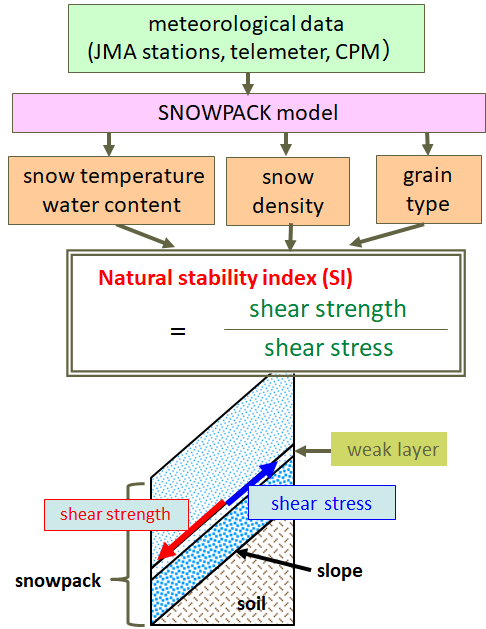
The natural stability index is used for natural avalanche prediction in Japan. The natural stability index is calculated as the ratio of shear strength to shear stress. Although avalanche risk is basically estimated using stability index, the cause of instability is estimated by other information. Information on grain type is used to estimate the cause of weak layer formation (e.g., timing of faceted crystals formation). Furthermore, information on the water content and discharge amount are required to estimate the wet snow avalanche risk. These data are included for the operation of avalanche prediction.
Examples of snowpack conditions with low stability
Figure (a) is the case for unstable conditions due to heavy snowfall. Fresh snow consolidates quickly after snowfall and usually strengthens enough to support newly fallen snow. However, if heavy snowfall continued for several hours, the snow is strongly loaded with newer snow before densification. In this case, snow becomes unstable even if there is no weak layer. After snowfall stops, the stability index increases rapidly.
In another case (Fig. b), the red line at about 200 cm above the ground surface indicates a weak layer (e.g., faceted crystals). This unstable condition continues for two days after cessation of snowfall. The main control schemes used to determine the stability index in SNOWPACK are the initial density of snow, compressive viscosity, and the relationship of snow density and shear strength.
Information on liquid water infiltration is used to estimate the wet snow full depth avalanche risk. The most recent version of the SNOWPACK model uses the Darcy?Buckingham law to simulate liquid water infiltration (Hirashima et al., 2010). Figure (c) shows an increase in water content during water percolation, which leads to increased wet snow avalanche risk.
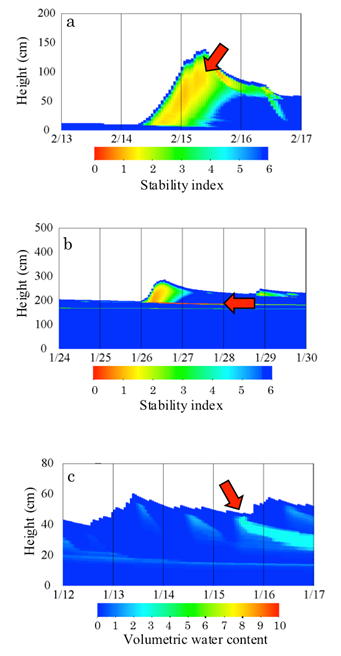
Improvements of the SNOWPACK model
Improvements have been made to SNOWPACK by SIRC/NIED, specifically to reflect the characteristics of snow observations in Japan. To express continuous variations of shear strength due to the transition process from rounded grains to faceted crystals, Hirashima et al. (2009) introduced the DSM factor in the expression of shear strength as a function of water vapor transport. To improve the scheme to calculate non-uniform water transportation in snow cover (preferential flow), cold room experiments were carried out, and the multi-dimensional water transport model was developed based on these results. A comparison of the laboratory results and those obtained from the water transport model is shown in the figure below. This experiment showed that liquid water ponded at the interface of fine over coarse snow layers due to the capillary barrier effect. This effect is reproduced by the water transport model fairly well.
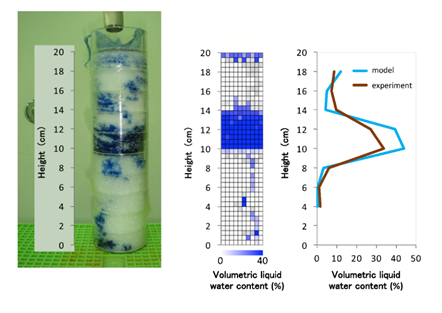
on the formation process of preferential flow.
See Hirashima et al. (2014, 2017) in detail of the improvement of avalanche warning.
- Hirashima, H., Abe, O., Sato, A. and Lehning, M. (2009): An adjustme nt for kinetic growth metamorphism to improve shear strength parameterization in the SNOWPACK model. Cold Reg. Sci. Technol., 59 (2-3), 169-177.
- Hirashima, H., S. Yamaguchi, and T. Katsushima, 2014: A multi-dimensional water transport model to reproduce preferential flow in the snowpack. Cold Reg. Sci. Technol., 108, 80-90.
- Hirashima, H., F. Avanzi, and S. Yamaguchi, 2017: Liquid water infiltration into a layered snowpack: evaluation of a 3-D water transport model with laboratory experiments. Hydrol. Earth Syst. Sci., 21(11), 5503-5515.